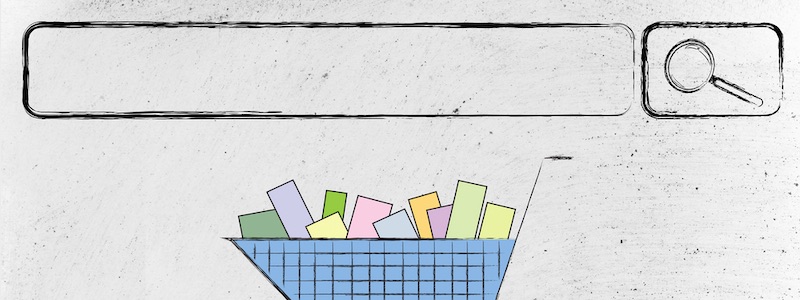
Modern search technology uses artificial intelligence and machine learning to deliver highly personalized and relevant results. The outcome is often a shorter path to purchase — and more conversions.
Retailers have greatly improved their online stores in the past few years. User flows are streamlined. Designs are better tuned to tell the brand’s story. Site speed is much faster. Stores are now optimized for mobile devices. Product content has been enhanced. Ratings and reviews are more credible and widely available.
But for many online stores, site search is weak. Consumers cannot find what they are looking for. The search returns too many products or items that do not fit the query. There may be five or more filters to narrow the search, but that means learning what the filters mean and deciding if they are likely to help.
Consumers are impatient. If they don’t find a product within a couple of search attempts, they will likely return to Google and click on the next best result.
Enhancing Site Search
By delivering more personalized and relevant product search results, you will improve your conversion rates and raise your average order value.
Most ecommerce platforms include a basic search engine. Merchants tend to use it until there’s a problem. Frequently the default search is based on Apache Lucene, an open-source search engine, which evolved into Solr, which then evolved into several other enhanced versions — Elastic, Lucidworks, others — that gave merchants better control over search results. Each iteration added capabilities but didn’t necessarily incorporate modern machine learning to improve the results.
There were also product-only search engines for ecommerce stores from SLI Systems and others that created their own indexes from a merchant’s product data feed. They used machine learning to improve results and offer predictive search queries. But many merchants were hesitant to pay for what they perceived as incremental revenue improvements. Merchants focused on other issues. Search was a low priority.
By delivering more personalized and relevant product search results, you will improve your conversion rates…
There are new entrants into the product search and recommendation space. Many come from enterprise search where the demands to index many sources of data drove them to develop powerful machine-learning algorithms that feed artificial-intelligence-powered results. Examples are Coveo and Algolia. Others, such as Bloomreach and Unbxd, are focused solely on delivering relevant search results as well as recommended products and accessories for cross-sells and upsells.
I recently attended a B2B ecommerce conference where the busiest vendor booths were search companies. Based on discussions I’ve had with large retailers, product search and recommendations are now a key focus. Better analytics are showing the dramatic drop-offs by consumers who are not finding the products they are searching for.
Retailers know that by creating a shorter path to purchase, consumers are more likely to consummate the transaction. Likewise, consumers are more likely to buy recommended accessories if they are truly relevant.
Personalization
There is much talk about personalization in ecommerce. Unique and relevant experiences — including search and recommendations — require machine learning. You cannot write rules for contextual personalization at scale, nor can you write rules to determine which products consumers are most likely to purchase.
To deliver relevant product search results, evaluate a searcher’s profile — how she found your site and what she purchased previously. What did other consumers purchase when they searched for similar items? What was their current click flow? Were they price sensitive? What about the time of day or month of the year? There are dozens of data points to consider.
When introduced in the mid-2000s, “predictive search” or “type-ahead search” helped consumers choose a phrase that the search engine seemed to understand. But the search results were still a long list of products, in no priority. Searches results were based on the text in the product descriptions and product names, not the intent of the query. Hence, if you searched for “blue jeans” the results might include every piece of blue clothing — not just “blue jeans.” This still occurs on many well-designed and popular ecommerce sites.
To deliver relevant product search results, evaluate a searcher’s profile…
Modern search technology is moving closer to ingesting all relevant data about a searcher and then delivering personalized, contextual results. Thus search results are the products that the buyer will likely purchase.
These machine-learning, AI-based search engines are API driven. You can easily drop them into your ecommerce platform with minimal disruption. They can power your web properties as well as mobile apps and voice searches.
They typically include features such as dynamic filtering from merchant-selected attributes. The result is often a shorter path to purchase.
Where to Start?
Search engine pricing varies based, typically, on the number of products and search queries, among other factors. Some search providers target large companies; others focus on smaller ones.
Evaluate the gaps in your current search results. Look at your analytics. Search for products yourself. Engage with search vendors. What’s involved with integrating their platform into your ecommerce site?
Remember, the fastest way to more ecommerce revenue is usually to increase the conversion rate. Invest in site search accordingly.